Machine Learning (ML) has rapidly become a cornerstone of technological advancement, enabling businesses across various sectors to harness data-driven insights and automate complex processes. As we move through 2024, the impact of ML solutions is more profound than ever, driving growth and innovation at an unprecedented pace. This article explores the multifaceted ways in which machine learning solutions are propelling growth in 2024, delving into specific industries, use cases, and future trends.
Table of Contents
Introduction
Machine learning, a subset of artificial intelligence (AI), involves the use of algorithms and statistical models to enable computers to perform tasks without explicit programming. By learning from data, these systems can identify patterns, make decisions, and improve over time. The applications of machine learning are vast, ranging from image and speech recognition to predictive analytics and autonomous systems.
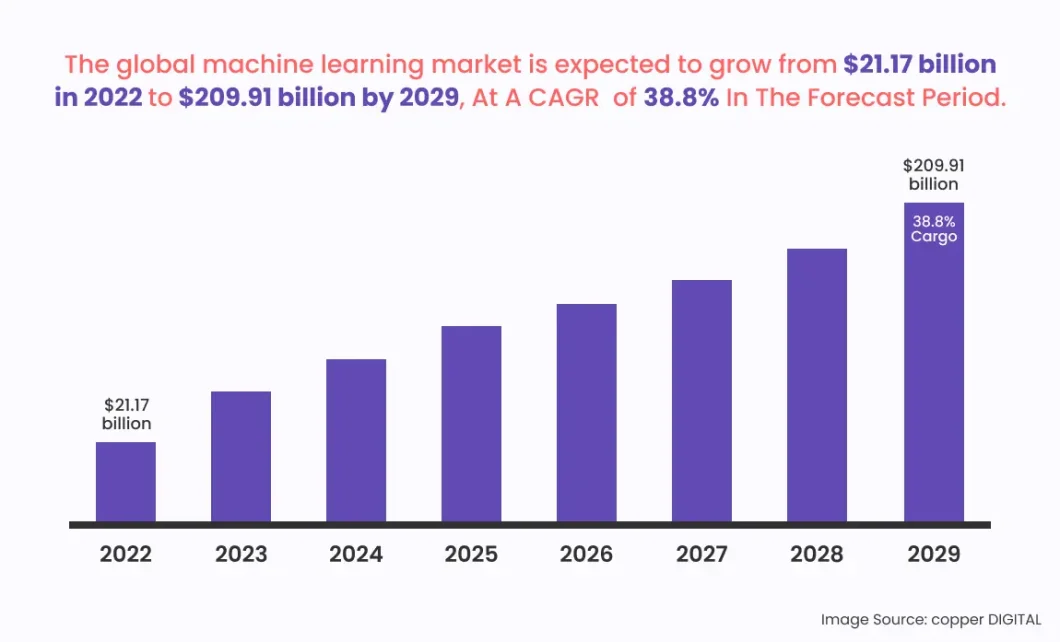
Key Statistics on Machine Learning Growth in 2024
- Global Market Size: The global machine learning market is projected to reach $21.17 billion in 2022 to $209.91billion by 2029, with a compound annual growth rate (CAGR) of 38.8% in the forecast period.
- Enterprise Adoption: Approximately 75% of enterprises are utilizing machine learning in 2024, up from 58% in 2021.
- Industry Impact:
- Healthcare: Machine learning in medical imaging is projected to reduce diagnostic errors by up to 50%.
- Finance: Financial institutions have reported a 30% reduction in fraudulent activities using ML.
- Emerging Trends: By 2024, 60% of AI applications are expected to incorporate explainable AI techniques, enhancing transparency and trust.
Key Components of Machine Learning
- Algorithms: The mathematical instructions that guide the learning process.
- Data: The raw material that the algorithms use to learn and make predictions.
- Models: The outputs of the learning process that can make predictions or decisions.
Machine Learning in Business Growth
Machine learning solutions are revolutionizing the way businesses operate, offering tools to enhance decision-making, improve operational efficiency, and deliver personalized experiences to customers.
Enhancing Decision Making
Machine learning algorithms analyze vast amounts of data to uncover patterns and insights that humans might miss. This capability allows businesses to make more informed decisions.
- Predictive Analytics: Using historical data to predict future trends.
- Real-time Analytics: Providing immediate insights for time-sensitive decisions.
- Data-Driven Strategy: Formulating business strategies based on robust data analysis.
Improving Operational Efficiency
Automation through machine learning reduces the need for manual intervention in repetitive tasks, leading to significant cost savings and efficiency improvements.
- Process Automation: Automating routine tasks such as data entry and report generation.
- Supply Chain Optimization: Predicting demand and managing inventory efficiently.
- Maintenance and Operations: Predictive maintenance to prevent equipment failures.
Customer Personalization
Machine learning enables businesses to offer highly personalized experiences to customers, enhancing satisfaction and loyalty.
- Recommendation Systems: Suggesting products or services based on customer behavior.
- Dynamic Pricing: Adjusting prices in real-time based on demand and competition.
- Customer Segmentation: Identifying distinct customer groups for targeted marketing.
Industry-Specific Applications of Machine Learning
The transformative power of machine learning is evident across various industries, each leveraging its capabilities to solve unique challenges and drive growth.
Healthcare
Machine learning is revolutionizing healthcare by improving diagnostics, personalizing treatment, and streamlining administrative tasks.
- Medical Imaging: Enhancing the accuracy of disease detection through image analysis.
- Predictive Analytics: Forecasting patient outcomes and optimizing treatment plans.
- Drug Discovery: Accelerating the process of developing new medications.
Finance
In the finance industry, machine learning algorithms are crucial for risk management, fraud detection, and personalized banking services.
- Fraud Detection: Identifying and preventing fraudulent activities in real-time.
- Credit Scoring: Assessing creditworthiness more accurately than traditional methods.
- Algorithmic Trading: Using predictive models to execute trades at optimal times.
Retail
Retailers use machine learning to enhance customer experiences, optimize inventory, and streamline supply chains.
- Personalized Marketing: Delivering targeted advertisements based on consumer behavior.
- Inventory Management: Predicting stock levels to meet customer demand without overstocking.
- Customer Service: Implementing chatbots and virtual assistants to improve service.
Manufacturing
Machine learning in manufacturing leads to improved quality control, predictive maintenance, and optimized production processes.
- Quality Control: Using image recognition to detect defects in products.
- Predictive Maintenance: Anticipating equipment failures before they occur.
- Production Optimization: Streamlining production lines for maximum efficiency.
Transportation and Logistics
Machine learning enhances the efficiency and reliability of transportation and logistics operations.
- Route Optimization: Finding the most efficient routes for delivery.
- Demand Forecasting: Predicting shipping volumes to optimize logistics.
- Autonomous Vehicles: Developing self-driving technology for safer transportation.
Emerging Trends in Machine Learning for 2024
As machine learning continues to evolve, several emerging trends are shaping its future and expanding its applications.
Explainable AI (XAI)
Explainable AI aims to make machine learning models more transparent and understandable to humans.
- Transparency: Allowing users to understand how decisions are made.
- Accountability: Ensuring that AI systems can be held accountable for their actions.
- Trust: Building trust in AI systems by making their operations clear.
Edge Computing
Edge computing involves processing data closer to where it is generated, reducing latency and bandwidth use.
- Real-time Processing: Enabling faster decision-making by processing data locally.
- Cost Efficiency: Reducing the need for expensive data transmission to central servers.
- Scalability: Allowing for more scalable and distributed AI applications.
Federated Learning
Federated learning enables multiple organizations to collaboratively train machine learning models without sharing their data.
- Privacy: Preserving data privacy by keeping data localized.
- Collaboration: Allowing organizations to benefit from shared learning.
- Efficiency: Reducing the need for large centralized datasets.
AutoML
AutoML (Automated Machine Learning) automates the process of applying machine learning to real-world problems.
- Accessibility: Making machine learning accessible to non-experts.
- Efficiency: Speeding up the development and deployment of ML models.
- Optimization: Automatically finding the best models and hyperparameters.
Challenges and Solutions in Implementing Machine Learning
While the benefits of machine learning are significant, implementing these solutions comes with its own set of challenges.
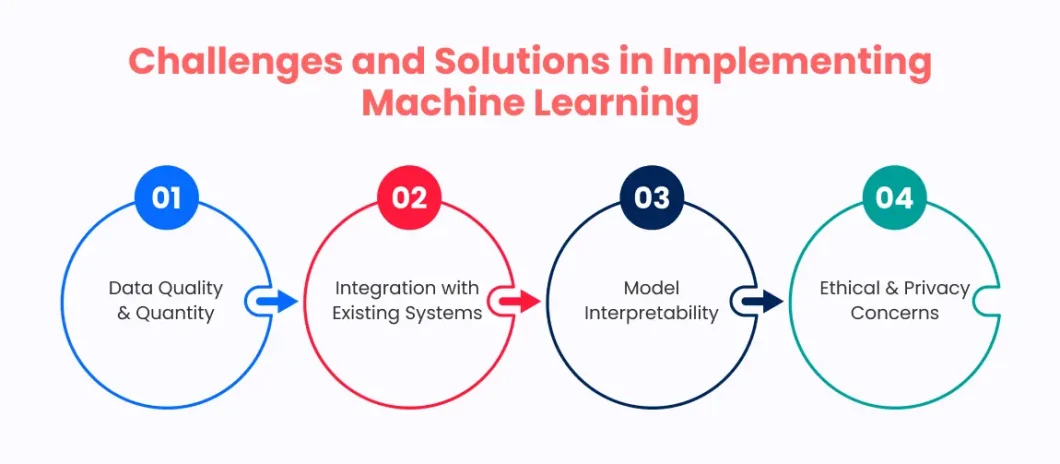
Data Quality and Quantity
High-quality and sufficient data are crucial for training effective machine learning models.
- Data Collection: Implementing robust data collection methods.
- Data Cleaning: Ensuring data is accurate and free from errors.
- Data Augmentation: Generating additional data to improve model performance.
Model Interpretability
Complex machine learning models can be difficult to interpret, making it hard to understand their decisions.
- Simpler Models: Using simpler models where interpretability is critical.
- Explainability Techniques: Applying techniques to explain complex models.
- User Education: Educating users about how models work and their limitations.
Integration with Existing Systems
Integrating machine learning solutions with existing IT infrastructure can be challenging.
- APIs: Using APIs to facilitate integration.
- Modular Design: Designing ML solutions to be modular and interoperable.
- Collaboration: Working closely with IT teams to ensure smooth integration.
Ethical and Privacy Concerns
The use of machine learning raises ethical and privacy issues that need to be addressed.
- Ethical Guidelines: Developing and adhering to ethical guidelines for AI use.
- Privacy Protection: Implementing strong privacy protection measures.
- Bias Mitigation: Ensuring models are free from bias and fair.
Case Studies of Machine Learning Driving Growth
Examining real-world case studies helps illustrate the impact of machine learning on business growth.
1: Healthcare
A leading healthcare provider implemented machine learning to improve patient diagnostics and treatment plans. By analyzing medical records and imaging data, the ML system could predict patient outcomes with high accuracy, leading to better treatment decisions and improved patient outcomes.
2: Finance
A major financial institution used machine learning to enhance its fraud detection capabilities. By analyzing transaction patterns in real-time, the system could detect and prevent fraudulent activities more effectively than traditional methods, saving the company millions of dollars annually.
3: Retail
A global retail chain leveraged machine learning to optimize its inventory management. By predicting customer demand and adjusting stock levels accordingly, the company reduced excess inventory and improved its supply chain efficiency, resulting in significant cost savings and increased customer satisfaction.
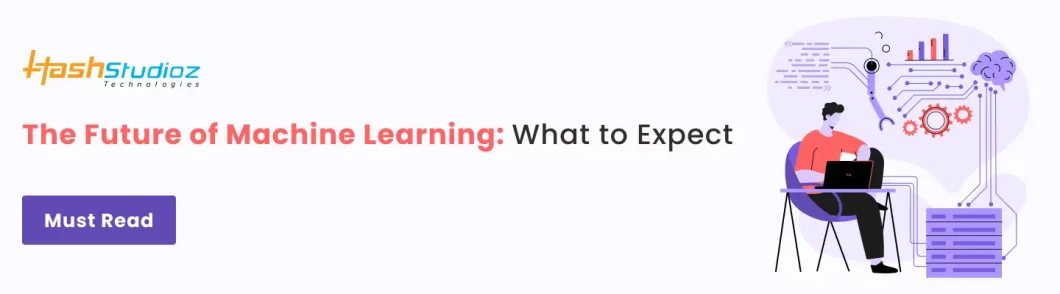
The Future of Machine Learning Solutions
The future of machine learning is bright, with continuous advancements and expanding applications. As more businesses recognize the potential of ML, its adoption will become more widespread, driving further innovation and growth.
- Continuous Learning: ML models that continuously learn and adapt to new data.
- AI and IoT Integration: Combining AI with Internet of Things (IoT) devices for smarter systems.
- Human-AI Collaboration: Enhancing collaboration between humans and AI for better outcomes.
Conclusion
Machine learning solutions are driving growth across various industries in 2024 by enhancing decision-making, improving operational efficiency, and providing personalized customer experiences. Despite the challenges, the benefits of implementing machine learning far outweigh the drawbacks, making it an essential tool for businesses looking to innovate and stay competitive. As technology continues to evolve, the role of machine learning in driving growth will only become more significant, shaping the future of industries and transforming the way we live and work.
HashStudioz Technologies is a cutting-edge software development company renowned for its innovative solutions in digital transformation and IT consultancy. Specializing in bespoke software development, web and mobile applications, and cloud services, HashStudioz leverages advanced technologies to deliver tailored solutions that meet the unique needs of businesses across various industries. With a commitment to quality and client satisfaction, HashStudioz Technologies continues to push the boundaries of technology to drive efficiency, growth, and success for its global clientele.
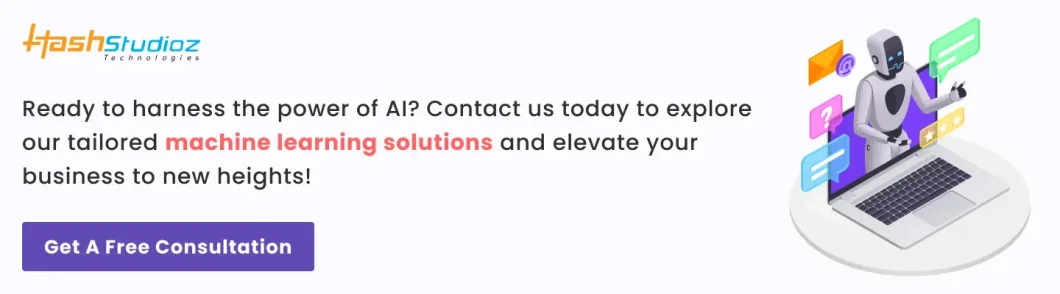