Self-driving cars are no longer just experimental prototypes. With rapid advancements in artificial intelligence (AI), these vehicles are becoming a significant part of the future of transportation. At the center of this transformation is AI in self-driving cars, which allows vehicles to perceive the environment, make decisions, and move with minimal human input.
Artificial intelligence is the foundation of self-driving vehicle technology. From real-time data processing to continuous learning, AI enables vehicles to perform complex driving tasks that once required human intelligence.
According to McKinsey & Company, autonomous vehicles could generate $300 billion to $400 billion in revenue by 2035. This growth would not be possible without the contributions of Generative AI Development Companies and Artificial Intelligence Services, which design the algorithms and systems powering this transformation.
Table of Contents
- Understanding AI in Self-Driving Cars
- Key Components of AI in Autonomous Vehicles
- The Role of a Generative AI Development Company
- How Generative AI Supports Self-Driving Technology
- Real-World Applications of Generative AI in Self-Driving Systems
- Artificial Intelligence Services Driving Innovation
- Applications of AI in Self-Driving Cars
- Technical Challenges in Self-Driving AI
- Partner with HashStudioz – Your AI Engineering Partner
- Conclusion
Understanding AI in Self-Driving Cars
AI in self-driving cars refers to the use of artificial intelligence algorithms to control, guide, and monitor autonomous vehicles. These systems rely on sensors, cameras, radar, and LiDAR to perceive surroundings and make driving decisions.
The AI system must process thousands of data points per second. It must analyze road conditions, recognize pedestrians, detect other vehicles, and respond in real time. This requires high-performance computing and machine learning techniques, especially deep learning and reinforcement learning.
Key Components of AI in Autonomous Vehicles
AI forms the brain of self-driving cars. It enables perception, decision-making, and control, all of which are essential for fully autonomous operations. These components work together to allow a vehicle to safely navigate roads, interpret surroundings, and react in real-time. Below is a technical breakdown of each core AI component in autonomous vehicles.
1. Perception Systems
What is Perception in Autonomous Vehicles?
Perception is the AI’s ability to see, understand, and interpret the environment around the vehicle. It collects and analyzes data from a range of onboard sensors and cameras.
Core Functions of Perception Systems:
- Object Detection: The AI identifies and classifies vehicles, pedestrians, cyclists, animals, and other objects on the road.
- Traffic Signal Recognition: Traffic lights and stop signs are recognized to follow legal road behavior.
- Lane Detection: Lane markings and road boundaries are detected to help with lane keeping and changing.
- Obstacle Detection: AI identifies static and dynamic obstacles and recalculates paths when necessary.
Technology Used:
- Cameras: Provide high-resolution visual data.
- LiDAR (Light Detection and Ranging): Measures distance using laser pulses and builds 3D maps of surroundings.
- Radar: Detects object velocity and distance, especially useful in poor visibility.
- Ultrasonic Sensors: Assist with close-range detections like parking or detecting curbs.
AI Models Used:
- Convolutional Neural Networks (CNNs): These are specialized for visual tasks like object detection, segmentation, and classification.
- Semantic Segmentation: This technique allows each pixel of an image to be classified into categories (e.g., road, car, pedestrian).
Example: Tesla’s Autopilot uses cameras with AI-based vision processing to detect road edges, cars, and lane markings without relying heavily on LiDAR.
2. Decision-Making Systems
Once the AI understands its surroundings through perception, it must make decisions about what to do next. This is where decision-making systems come in.
Key Decision-Making Functions:
- Path Planning: Determines the safest and most efficient route based on the vehicle’s goal.
- Behavior Prediction: AI predicts what other drivers, pedestrians, or cyclists will do next. For example, if someone is about to jaywalk.
- Action Selection: The AI decides whether to turn, brake, accelerate, or stop, depending on the context.
- Handling Uncertainty: AI must respond to unpredictable events like a pedestrian running into the street.
AI Algorithms Used:
- Reinforcement Learning: Models learn optimal decisions through trial and error in simulated environments. They get better over time with more experience.
- Markov Decision Processes (MDPs): Help model the probabilities of different outcomes and choose the best action sequence.
- Behavior Cloning: Trains AI to mimic human driving behavior by learning from real driving data.
Example: Waymo uses a decision-making stack that includes prediction models and trajectory planners to decide the vehicle’s next actions while anticipating what others might do.
Train Reservation Software: Benefits for Operators and Passengers
3. Control Systems
Once the AI decides on a course of action, it must translate that decision into movement. This is where control systems operate.
Core Control Functions:
- Steering Control: Adjusts the wheel angle to maintain the desired trajectory.
- Acceleration: Increases speed when safe and necessary.
- Braking: Slows down or stops the vehicle, factoring in distance to objects and traffic signals.
- Adaptive Cruise Control: Maintains a set speed and distance from the vehicle in front.
Key Requirements:
- Precision: The AI must make accurate micro-adjustments in real time to stay within lanes and maintain safety.
- Speed: Decisions must be executed in milliseconds to avoid accidents.
- Redundancy: Safety mechanisms must exist in case of sensor failure or software bugs.
AI Techniques in Control:
- Model Predictive Control (MPC): A method that anticipates future states of the vehicle and adjusts control actions accordingly.
- Neural Network Controllers: These can learn from experience to improve control decisions over time.
- Feedback Loops: The system continually compares expected vs. actual outcomes and adjusts actions in real time.
Example: NVIDIA’s Drive platform uses AI-based controllers that handle multiple driving tasks while adjusting to feedback from sensors to ensure stability and accuracy.
The Role of a Generative AI Development Company
A Generative AI Development Company plays a critical role in advancing machine intelligence by designing and developing algorithms that can produce human-like content and simulations. These companies focus on creating AI models that not only understand existing data but also generate new, meaningful data. This capability is particularly useful in industries like autonomous driving, where safety, accuracy, and performance depend heavily on robust training datasets.
How Generative AI Supports Self-Driving Technology
Self-driving vehicles rely on AI systems trained through thousands of hours of data. However, collecting enough real-world data for every possible scenario is nearly impossible. This is where a Generative AI Development Company steps in, offering solutions that simulate and augment data effectively.
1. Scenario Simulation for Training AI Models
Generative AI companies build tools to simulate realistic driving situations. These scenarios include a wide range of possibilities:
- Pedestrian behavior at intersections
- Lane-switching vehicles
- Emergency vehicles on the road
- Sudden changes in traffic flow
By simulating these events in a controlled environment, self-driving car developers can test how their models react. These simulations help identify weaknesses before the cars hit public roads.
2. Data Augmentation to Improve Model Robustness
Collecting enough diverse data can be time-consuming and expensive. Generative AI helps solve this problem by creating augmented datasets from existing ones. For example:
- Generating new images by altering weather, lighting, and angles
- Modifying road layouts or traffic conditions
- Adding or removing road objects like cones or signs
This data helps AI systems learn to handle a variety of conditions, improving performance in the real world.
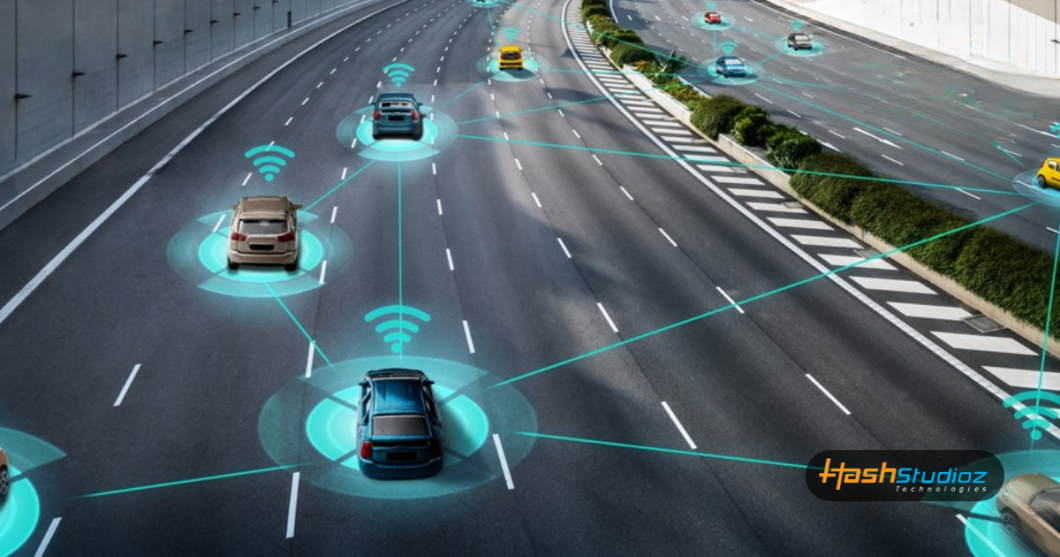
3. Synthetic Environment Creation for Edge Case Testing
Edge cases are rare events that may not appear in typical datasets. These can include:
- A child suddenly running into the road
- Vehicles breaking traffic rules
- Fog reducing visibility to near zero
A Generative AI Development Company can create synthetic environments to test such edge cases without putting real people or vehicles in danger. These simulations replicate complex visual and behavioral conditions that would be difficult to capture otherwise.
Real-World Applications of Generative AI in Self-Driving Systems
Generative AI is playing a major role in the development and testing of autonomous vehicles. Several leading companies use this technology to improve model accuracy, test vehicles in rare conditions, and reduce the need for real-world driving miles. These companies rely on Generative AI Development Services to create realistic training environments and synthetic data.
1. Waymo: Simulating Driving at Scale
Waymo, a leader in self-driving technology, uses simulation to test and improve its autonomous vehicles. The company runs over 20 million miles of virtual driving per day using its simulator. Generative AI plays a key role in creating realistic traffic scenarios that mimic actual city conditions. These include unpredictable events like jaywalking pedestrians, aggressive drivers, or sudden roadblocks. This helps Waymo identify and fix issues before testing cars on public roads.
2. Tesla: Constant Learning Through Simulation
Tesla uses data from its fleet of cars to improve its Full Self-Driving (FSD) software. It combines real-world driving data with simulation powered by generative AI. These tools recreate challenging situations that vehicles might not encounter often in actual use. Examples include:
- Unusual intersections
- Poorly marked lanes
- Pedestrian-heavy environments
Tesla’s AI team uses synthetic data to simulate these edge cases. The system then retrains itself based on model performance in these simulations.
3. NVIDIA Drive Sim: Synthetic Worlds for Training
NVIDIA’s Drive Sim is a simulation platform powered by generative AI. It allows developers to test self-driving systems in fully synthetic environments. The tool can simulate:
- Traffic flow changes
- Different lighting and weather conditions
- Road surface textures and colors
Drive Sim uses real-time physics and AI-generated content to create highly realistic environments. These environments help validate vehicle software in every possible situation.
4. Cruise: City-Scale Simulation
Cruise, backed by General Motors, uses generative AI to test autonomous vehicles in digital copies of cities like San Francisco. These virtual environments include:
- Realistic building structures
- Accurate traffic signals and signs
- Human behavior models for pedestrians and drivers
Using synthetic environments, Cruise trains its AI systems to respond to dense urban traffic. This is safer and quicker than relying on real-world testing alone.
Artificial Intelligence Services Driving Innovation
Artificial Intelligence Services refer to end-to-end solutions provided by companies to build, deploy, and maintain AI systems. In the self-driving industry, these services include:
Certainly! Below is a detailed explanation for each point from Artificial Intelligence Services Driving Innovation, with each description in 60 words:
1. AI Model Development
AI model development involves designing and training algorithms that can detect, classify, and make driving decisions in real time. This includes object recognition, lane detection, and path planning. These models form the foundation of autonomous driving systems, ensuring vehicles respond correctly to various road situations through continuous learning and performance improvements using large-scale driving datasets.
2. Data Labeling and Annotation
Data labeling is the process of tagging objects like cars, pedestrians, traffic signs, and road lanes in images and videos. This step is essential to train supervised learning models. Accurate annotation ensures that AI systems can detect and react to their environment effectively. It also improves safety and model accuracy during both training and real-world deployment.
3. Real-time Inference Optimization
Inference optimization involves reducing the time it takes for AI models to make decisions while driving. Techniques include model pruning, quantization, and using efficient architectures. This is vital for self-driving vehicles where even milliseconds count. Optimized inference ensures smoother navigation, quicker responses to hazards, and reduced computational load on the vehicle’s hardware systems.
4. Edge Deployment and Integration
Edge deployment refers to installing AI models directly on in-vehicle systems rather than relying on cloud servers. This enables real-time decision-making without latency. Integration ensures that AI modules work seamlessly with sensors, cameras, and control units. It improves system reliability, especially in areas with poor connectivity, and supports continuous vehicle operation under demanding road conditions.
5. Compliance with Safety Standards
AI systems in vehicles must follow regulations like ISO 26262 to ensure functional safety. This includes validating software behavior, running risk assessments, and documenting development steps. Meeting these standards builds trust and allows companies to deploy their vehicles commercially. It also protects passengers and pedestrians by ensuring predictable and well-tested vehicle behavior in real traffic.
6. Explainable AI Models
Explainable AI focuses on making model decisions understandable to humans. In self-driving systems, this helps engineers, regulators, and users interpret why the vehicle made a certain choice. This transparency improves debugging, boosts user confidence, and ensures the technology meets ethical and legal standards. It’s especially important when vehicles are involved in high-stakes or emergency scenarios.
AI services also involve compliance with safety standards like ISO 26262 for functional safety and the development of explainable AI models for better transparency.
Applications of AI in Self-Driving Cars
1. Traffic Sign Recognition
AI models are trained on large datasets of traffic sign images to recognize road signs with high accuracy. These systems identify speed limits, stop signs, yield signs, and temporary warnings, helping vehicles follow laws and react to changes on the road. This real-time recognition is crucial for ensuring passenger safety and maintaining legal compliance during autonomous driving.
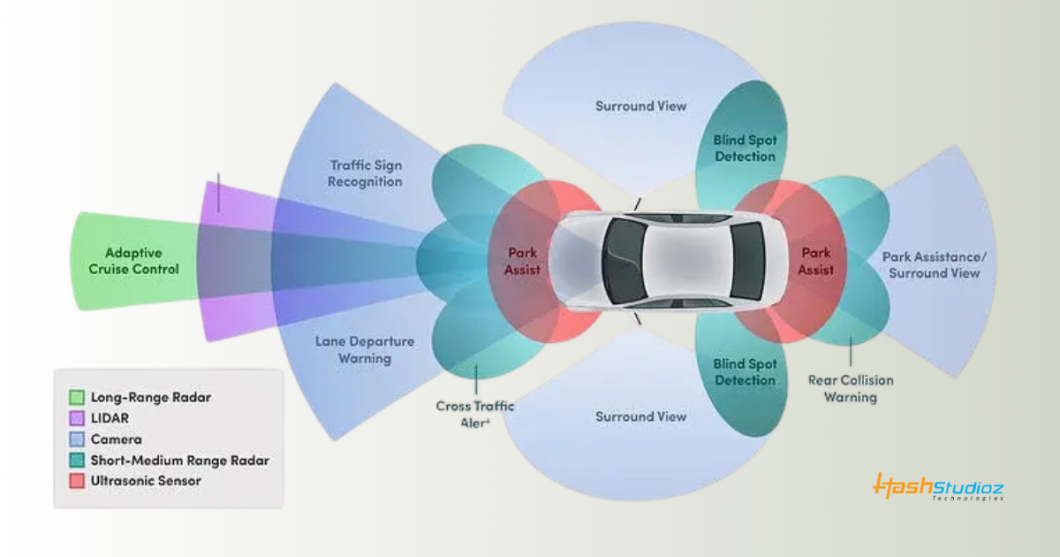
2. Lane Keeping
Self-driving cars use AI-driven computer vision to detect and follow lane markings. The system keeps the vehicle centered, assists with safe lane changes, and prevents unintentional lane departures. According to the National Highway Traffic Safety Administration (NHTSA), lane departure warning systems can reduce single-vehicle, sideswipe, and head-on crashes by up to 21%, improving overall road safety.
3. Collision Avoidance
AI processes data from sensors like LiDAR, radar, and cameras to detect surrounding objects and calculate the time to collision. This enables quick responses such as automatic emergency braking, detecting pedestrians or cyclists, and tracking moving vehicles. Systems from Tesla and Waymo rely on such technologies to reduce human error and react instantly to avoid potential accidents.
4. Route Optimization
AI helps plan the best possible route using live traffic conditions, road closures, and vehicle capabilities like fuel or battery range. It adjusts paths to avoid congested areas and delays, reducing travel time and fuel usage. This is especially beneficial for autonomous delivery or ride-hailing fleets where efficiency directly impacts operational cost and customer satisfaction.
Technical Challenges in Self-Driving AI
Despite progress, there are several technical challenges in AI in self-driving cars:
1. Edge Case Detection
AI systems struggle with rare and unpredictable scenarios, such as a pedestrian suddenly crossing the road in an unusual outfit or an animal darting across. These “edge cases” are not well represented in training data. Handling such anomalies requires massive datasets, simulation-based testing, and continual model learning to ensure safe and adaptive driving behavior.
2. Sensor Fusion
Self-driving vehicles rely on multiple sensors, including radar, LiDAR, and cameras. Combining this data accurately—called sensor fusion—is complex. Each sensor type has different strengths and weaknesses, and aligning their outputs in real-time for accurate perception of surroundings is a challenge. Proper fusion is vital to reduce blind spots and improve situational awareness for autonomous systems.
3. Low-light and Weather Conditions
AI vision systems often underperform in conditions like fog, heavy rain, snow, or nighttime. Cameras may lose visibility, and LiDAR or radar data can be distorted or obstructed. These limitations make it hard for AI to detect road edges, objects, or signs. Enhancing perception in adverse conditions requires better sensor calibration and more robust AI models.
4. Real-time Inference
Self-driving AI must make decisions in milliseconds. The vehicle continuously processes vast data from multiple sensors, maps, and traffic rules. Delays in inference can lead to unsafe situations. Building lightweight, optimized models and deploying them on edge computing hardware is essential for real-time performance and avoiding latency that could compromise safety.
5. Ethical and Legal Decisions
AI may face emergency scenarios where it must choose between two harmful outcomes—known as ethical dilemmas. Deciding whether to protect the passenger or pedestrians raises complex moral and legal questions. Programming such value-based decision-making into AI is highly controversial and still lacks universal guidelines or regulation, posing a significant challenge for autonomous development.
Partner with HashStudioz – Your AI Engineering Partner
At HashStudioz, we specialize in advanced Artificial Intelligence Services and Generative AI Development tailored for the autonomous vehicle industry. From building real-time AI models to creating simulation tools for edge-case testing, we deliver technical precision and scalable solutions.
Whether you are building autonomous delivery robots, self-driving trucks, or city taxis, our team helps you develop safe, intelligent, and regulation-ready systems.
Let’s build the future of AI in transportation together. Contact HashStudioz today for a technical consultation.
Conclusion
AI in self-driving cars is transforming the future of transportation. From perception to control, every core function of an autonomous vehicle depends on AI technologies. Generative AI Development Companies and providers of Artificial Intelligence Services continue to push the boundaries by enabling more accurate, efficient, and safer driving systems.
As the industry moves forward, improvements in simulation, data processing, and decision-making will determine the pace of adoption. The combination of AI research, industrial expertise, and scalable software solutions is what will ultimately bring safe autonomous vehicles to the roads worldwide.