The convergence of Artificial Intelligence (AI) and the Internet of Things (IoT) is revolutionizing the industrial landscape. These two technological forces are driving significant changes in how industries operate, optimize, and innovate their production processes.
From enhancing efficiency and reducing downtime to enabling predictive maintenance and improving quality control, AI and IoT are reshaping the manufacturing sector and other industries reliant on complex production processes.
In this post, we will look at the main areas where AI and IoT are having a significant impact on industrial production as we examine how these technologies are changing it. We’ll talk about how AI and IoT are integrated and how they help with supply chain optimization, quality assurance, predictive maintenance, energy management, and other areas.
We will also look at the opportunities and problems that arise from the use of these technologies, giving us a thorough grasp of how they will affect industry going forward.
Table of Contents
- The Integration of AI and IoT in Industrial Production
- Predictive Maintenance: Reducing Downtime and Costs
- Optimizing Supply Chain Management with AI and IoT
- Enhancing Quality Control and Assurance
- Energy Management and Sustainability
- Challenges and Opportunities in the Adoption of AI and IoT
- Future Trends in AI and IoT in Industrial Production
- The Role of Human Workers in the AI and IoT-Driven Industry
- The Evolution of AI and IoT Technologies
- Leading Industrial IoT Platform Providers: Transforming Industry Through Connectivity and Innovation
- Conclusion:
The Integration of AI and IoT in Industrial Production
Understanding AI and IoT
Artificial Intelligence (AI) refers to the simulation of human intelligence in machines. These machines are designed to think, learn, and perform tasks that typically require human intelligence, such as decision-making, pattern recognition, and problem-solving. AI can be categorized into various subfields, including machine learning, deep learning, natural language processing, and computer vision.
The Internet of Things (IoT) is a network of interconnected devices that communicate and share data with each other. These devices, embedded with sensors, software, and other technologies, collect and exchange data over the internet, enabling real-time monitoring, analysis, and control. IoT devices range from simple sensors to complex industrial machines.
The Synergy of AI and IoT
The combination of AI and IoT, often referred to as AIoT, creates a powerful synergy that enhances the capabilities of both technologies. IoT devices generate vast amounts of data from industrial processes, which can be analyzed and interpreted by AI algorithms to derive actionable insights. This integration enables industries to automate processes, optimize operations, and make data-driven decisions.
For instance, in a manufacturing plant, IoT sensors can monitor machine performance in real-time, while AI algorithms analyze the data to predict potential failures and suggest maintenance actions. This proactive approach not only reduces downtime but also extends the lifespan of machinery, leading to cost savings and improved productivity.
The Role of Edge Computing in AIoT
Edge computing plays a crucial role in the AIoT ecosystem by processing data closer to the source, reducing latency and bandwidth usage. In industrial environments, where real-time decision-making is critical, edge computing ensures that data from IoT devices is processed locally, allowing for faster response times. This is particularly important in scenarios where milliseconds can make a difference, such as in automated quality control or safety monitoring.
By combining edge computing with AI and IoT, industries can achieve real-time analytics and decision-making, leading to more efficient and agile production processes.
Predictive Maintenance: Reducing Downtime and Costs
The Challenge of Unplanned Downtime
Unplanned downtime is one of the most significant challenges faced by industries, leading to costly disruptions in production and delays in delivery schedules. Traditional maintenance practices, such as reactive and preventive maintenance, often fall short in addressing this issue. Reactive maintenance responds to equipment failures after they occur, while preventive maintenance is based on predefined schedules, regardless of the actual condition of the equipment.
How AI and IoT Enable Predictive Maintenance
Predictive maintenance leverages AI and IoT to predict when equipment is likely to fail, allowing maintenance teams to perform repairs before a breakdown occurs. IoT sensors continuously monitor the health and performance of machinery, collecting data on temperature, vibration, pressure, and other critical parameters. AI algorithms analyze this data to identify patterns and anomalies that indicate potential failures.
For example, a machine that starts to vibrate abnormally could signal an impending bearing failure. AI can detect this anomaly and notify the maintenance team to inspect and replace the bearing before it causes a complete breakdown. This proactive approach not only minimizes downtime but also reduces maintenance costs by preventing catastrophic failures.
Benefits of Predictive Maintenance
The adoption of predictive maintenance offers several benefits to industries:
- Reduced Downtime: By addressing issues before they lead to equipment failure, predictive maintenance significantly reduces unplanned downtime.
- Cost Savings: Preventing major breakdowns and optimizing maintenance schedules lead to cost savings in repairs and replacement parts.
- Extended Equipment Lifespan: Regular monitoring and timely maintenance extend the lifespan of machinery and equipment.
- Improved Safety: Identifying potential failures early reduces the risk of accidents and enhances workplace safety.
Case Studies of Predictive Maintenance in Industries
Several industries have successfully implemented predictive maintenance strategies powered by AI and IoT:
- Automotive Manufacturing: In automotive plants, predictive maintenance is used to monitor the condition of assembly line robots and other critical equipment. By predicting and preventing failures, manufacturers can maintain high production rates and meet delivery deadlines.
- Oil and Gas Industry: In the oil and gas sector, predictive maintenance is employed to monitor drilling rigs, pipelines, and compressors. Early detection of issues helps prevent costly disruptions in production and ensures continuous operation.
- Energy Sector: Power plants use predictive maintenance to monitor turbines, generators, and transformers. This ensures reliable energy production and reduces the risk of blackouts.
Optimizing Supply Chain Management with AI and IoT
The Complexity of Modern Supply Chains
Modern supply chains are complex, involving multiple stakeholders, global sourcing, and intricate logistics networks. Managing these supply chains efficiently is crucial for industries to maintain competitiveness, meet customer demands, and minimize costs. However, traditional supply chain management practices often struggle to cope with the dynamic nature of global markets and the increasing demand for agility and responsiveness.
The Role of AI and IoT in Supply Chain Optimization
AI and IoT are transforming supply chain management by providing real-time visibility, predictive insights, and automation. IoT devices, such as GPS trackers, RFID tags, and smart sensors, collect data at every stage of the supply chain, from raw material procurement to product delivery. AI algorithms analyze this data to optimize inventory levels, predict demand, and enhance logistics operations.
Real-Time Inventory Management
One of the key applications of AI and IoT in supply chain management is real-time inventory management. IoT-enabled sensors track the movement and status of inventory in warehouses and distribution centers. AI algorithms analyze this data to optimize stock levels, ensuring that products are available when needed while minimizing excess inventory.
For instance, AI can predict demand based on historical sales data, market trends, and external factors such as weather conditions. This enables industries to adjust their inventory levels in real-time, reducing the risk of stockouts or overstocking.
Enhancing Logistics and Transportation
Logistics and transportation are critical components of the supply chain that can benefit significantly from AI and IoT integration. IoT devices, such as GPS trackers and telematics systems, provide real-time data on the location and condition of goods in transit. AI algorithms analyze this data to optimize routes, reduce fuel consumption, and improve delivery times.
For example, AI can identify traffic patterns and recommend alternative routes to avoid delays, ensuring that goods reach their destination on time. Additionally, IoT sensors can monitor the condition of perishable goods, such as temperature-sensitive pharmaceuticals or food products, during transportation. If a deviation from optimal conditions is detected, AI can trigger corrective actions to prevent spoilage.
Predictive Analytics for Demand Forecasting
Accurate demand forecasting is essential for optimizing supply chain operations. AI-driven predictive analytics use historical data, market trends, and external factors to forecast future demand with high accuracy. This allows industries to align their production schedules, inventory levels, and procurement strategies with anticipated demand.
For instance, an AI model can predict an increase in demand for a particular product during a holiday season, enabling manufacturers to ramp up production and suppliers to ensure timely delivery of raw materials. This proactive approach minimizes the risk of stockouts, reduces lead times, and enhances customer satisfaction.
Case Studies of Supply Chain Optimization with AI and IoT
Several industries have leveraged AI and IoT to optimize their supply chain management:
- Retail Industry: Retailers use AI and IoT to manage inventory levels, predict demand, and optimize logistics. This ensures that products are available on store shelves when customers need them, while minimizing excess stock and reducing waste.
- Pharmaceutical Industry: The pharmaceutical sector uses AI and IoT to track the movement of drugs through the supply chain, ensuring compliance with regulations and maintaining product integrity. Predictive analytics help in managing inventory and reducing the risk of drug shortages.
- Automotive Industry: Automotive manufacturers use AI and IoT to optimize their global supply chains, ensuring the timely delivery of parts and components to assembly plants. Real-time visibility and predictive insights enhance production planning and reduce lead times.
24 Game-Changing IoT Innovations Transforming Our Daily Lives
Enhancing Quality Control and Assurance
The Importance of Quality Control in Industrial Production
Quality control is a critical aspect of industrial production, ensuring that products meet specified standards and customer expectations. Poor quality control can lead to product defects, recalls, and damage to a company’s reputation. Traditional quality control methods often rely on manual inspection, which can be time-consuming and prone to human error.
How AI and IoT Revolutionize Quality Control
AI and IoT are transforming quality control by enabling automated, real-time inspection and monitoring of production processes. IoT sensors collect data on various parameters, such as temperature, pressure, and humidity, during the manufacturing process. AI algorithms analyze this data to detect deviations from standard operating conditions that could lead to defects.
For example, in a production line for electronic components, IoT sensors can monitor soldering temperatures. If the temperature deviates from the optimal range, AI can identify the issue and alert operators to take corrective action, preventing defects in the final product.
Automated Visual Inspection
One of the most significant advancements in quality control is automated visual inspection using AI and computer vision. Traditional visual inspection methods rely on human inspectors to identify defects, which can be inconsistent and limited by human perception. AI-powered computer vision systems, on the other hand, can analyze images of products with high precision and consistency.
For instance, in the automotive industry, AI-powered cameras can inspect car body panels for dents, scratches, and other imperfections. These systems can identify defects that may be missed by human inspectors, ensuring that only high-quality products reach the market.
Predictive Quality Analytics
AI and IoT also enable predictive quality analytics, which involves using historical data to predict potential quality issues before they occur. By analyzing data from previous production runs, AI can identify patterns that correlate with defects and suggest process adjustments to prevent them.
For example, if a particular batch of raw material has been associated with a higher defect rate in the past, AI can flag this material and recommend alternative sources. This proactive approach reduces the risk of defects and ensures consistent product quality.
Case Studies of AI and IoT in Quality Control
Industries across various sectors have implemented AI and IoT for quality control:
- Electronics Manufacturing: In electronics manufacturing, AI and IoT are used to monitor the soldering process, ensuring that connections are made correctly and consistently. Automated visual inspection systems identify defects in circuit boards, reducing the risk of product failures.
- Food and Beverage Industry: The food and beverage industry uses AI and IoT to monitor production processes, ensuring that products meet safety and quality standards. For example, AI can analyze data from temperature sensors in a food processing plant to ensure that products are cooked or stored at the correct temperatures.
- Aerospace Industry: In the aerospace industry, AI-powered visual inspection systems are used to examine aircraft components for defects, ensuring that they meet stringent safety and quality requirements.
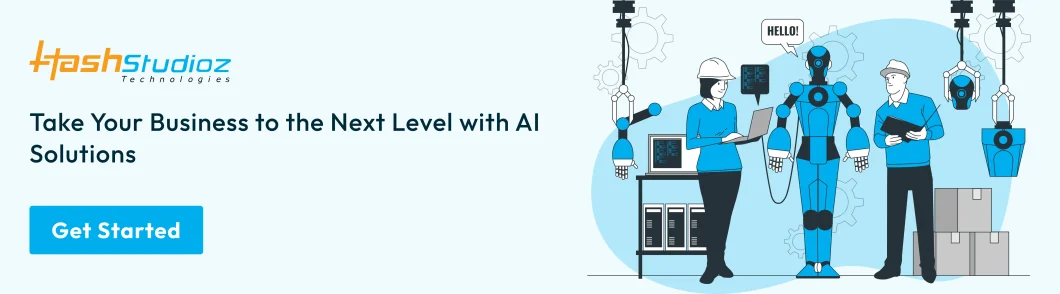
Energy Management and Sustainability
The Need for Energy Efficiency in Industry
Energy consumption is a significant operational cost for industries, and the demand for energy is expected to continue rising as production scales up. At the same time, there is increasing pressure to reduce carbon emissions and adopt sustainable practices. Achieving energy efficiency while maintaining production levels is a key challenge for industries.
AI and IoT in Energy Management
AI and IoT are playing a crucial role in helping industries manage their energy consumption more effectively. IoT sensors can monitor energy usage across various processes and equipment in real-time. AI algorithms analyze this data to identify inefficiencies, predict energy demand, and suggest optimization strategies.
For example, AI can analyze the energy consumption patterns of a manufacturing plant and recommend adjustments to operating schedules or equipment settings to reduce energy usage during peak hours. IoT sensors can also detect anomalies in energy consumption, such as a machine using more power than usual, indicating a potential fault that needs to be addressed.
Renewable Energy Integration
As industries increasingly turn to renewable energy sources, such as solar and wind, AI and IoT are essential for managing the integration of these sources into the energy mix. AI algorithms can predict the availability of renewable energy based on weather forecasts and historical data, allowing industries to optimize their energy consumption accordingly.
For instance, if AI predicts a sunny day with high solar energy generation, a manufacturing plant can schedule energy-intensive processes during daylight hours to maximize the use of solar power. This reduces reliance on fossil fuels and lowers carbon emissions.
Smart Grid and Demand Response
AI and IoT are also enabling the development of smart grids and demand response programs. Smart grids use IoT sensors and AI to monitor and manage the distribution of electricity in real-time. This ensures that energy is delivered efficiently and that supply matches demand.
Demand response programs, supported by AI and IoT, allow industries to adjust their energy consumption in response to signals from the grid. For example, during times of high electricity demand, AI can automatically reduce the energy usage of non-essential processes, helping to stabilize the grid and reduce costs.
Case Studies of Energy Management with AI and IoT
Industries are increasingly adopting AI and IoT for energy management and sustainability:
- Manufacturing Industry: In manufacturing, AI and IoT are used to monitor energy consumption and optimize production schedules to reduce energy costs. For example, a plant may use AI to schedule energy-intensive processes during off-peak hours when electricity rates are lower.
- Chemical Industry: The chemical industry uses AI and IoT to optimize the operation of energy-intensive processes, such as distillation and refining. AI-driven analytics identify opportunities to improve energy efficiency and reduce greenhouse gas emissions.
- Smart Buildings: In commercial and industrial buildings, AI and IoT are used to monitor and control heating, ventilation, and air conditioning (HVAC) systems, lighting, and other energy-consuming systems. This ensures optimal energy usage and reduces the carbon footprint.
Challenges and Opportunities in the Adoption of AI and IoT
Challenges in Implementing AI and IoT
While AI and IoT offer significant benefits, their adoption in industrial production is not without challenges:
- Data Security and Privacy: The vast amount of data generated by IoT devices raises concerns about data security and privacy. Industries must implement robust cybersecurity measures to protect sensitive information from breaches and unauthorized access.
- Integration with Legacy Systems: Many industries rely on legacy systems that may not be compatible with modern AI and IoT technologies. Integrating these systems can be complex and require significant investment.
- Skill Gaps: The successful implementation of AI and IoT requires a workforce with specialized skills in data science, machine learning, and IoT technology. Industries may face challenges in recruiting and training employees with the necessary expertise.
- Initial Costs: The initial investment required for AI and IoT implementation, including the cost of sensors, software, and infrastructure, can be a barrier for some industries. However, the long-term benefits often outweigh these costs.
Opportunities for Industries
Despite the challenges, the adoption of AI and IoT presents numerous opportunities for industries:
- Competitive Advantage: Industries that successfully implement AI and IoT gain a competitive advantage by improving efficiency, reducing costs, and enhancing product quality.
- Innovation and New Business Models: AI and IoT enable the development of new business models, such as predictive maintenance services and smart product offerings, opening up new revenue streams.
- Sustainability: The use of AI and IoT for energy management and waste reduction supports sustainability initiatives, helping industries meet regulatory requirements and enhance their corporate social responsibility.
- Global Collaboration: AI and IoT facilitate global collaboration by enabling remote monitoring and management of production processes across different locations. This supports the expansion of global supply chains and increases operational flexibility.
Future Trends in AI and IoT in Industrial Production
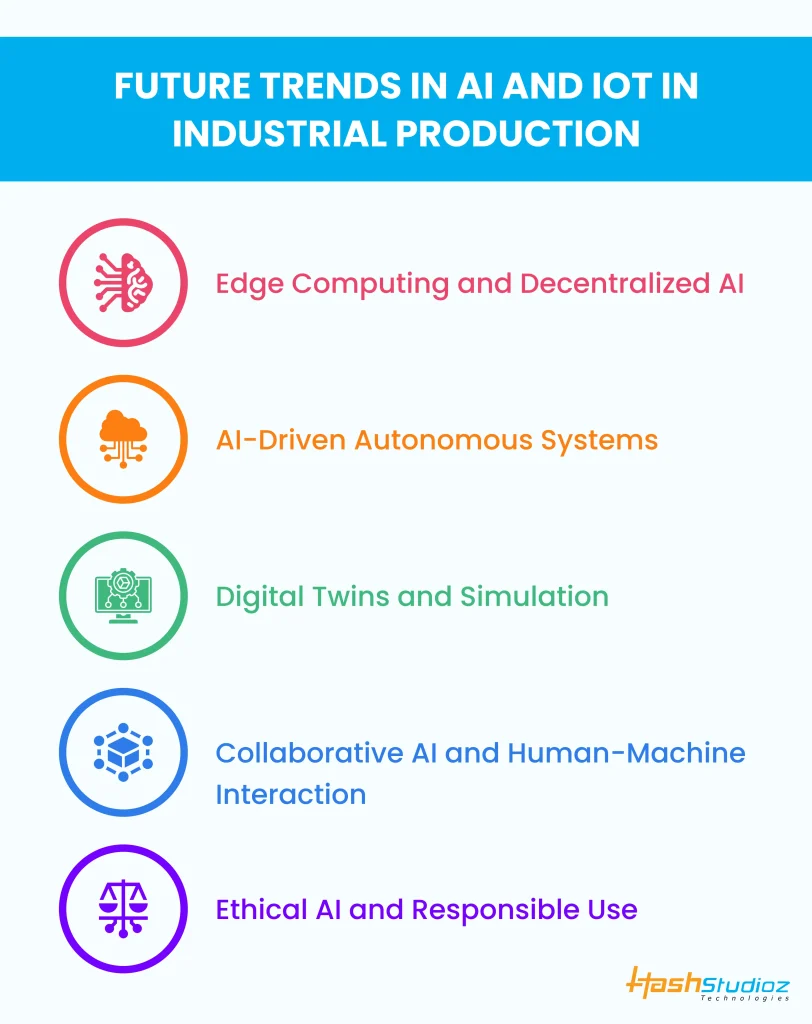
Edge Computing and Decentralized AI
As the number of IoT devices increases, the demand for real-time data processing is growing. Edge computing is emerging as a solution by processing data closer to where it is generated, rather than relying solely on centralized cloud servers. This trend is particularly relevant in industrial environments where real-time decision-making is crucial.
Edge computing enables AI algorithms to be deployed directly on IoT devices or local servers, reducing latency and ensuring faster responses. For example, in a smart factory, edge devices can process data from sensors on the production line to detect anomalies and trigger immediate corrective actions.
Decentralized AI, which distributes AI processing across multiple nodes rather than a central server, is another trend gaining traction. This approach enhances scalability, reduces bottlenecks, and increases resilience, especially in large-scale industrial environments.
AI-Driven Autonomous Systems
The future of industrial production may see a rise in AI-driven autonomous systems that can operate with minimal human intervention. These systems will be capable of learning from their environment, making decisions, and optimizing processes on their own.
For instance, autonomous robots equipped with AI can manage entire production lines, from raw material handling to packaging finished products. These robots can adapt to changing conditions, such as variations in raw material quality, and continuously improve their performance through machine learning.
In logistics, AI-powered autonomous vehicles and drones can transport goods within and between facilities, optimizing routes and reducing delivery times. These technologies will be crucial in creating fully automated supply chains.
Digital Twins and Simulation
Digital twins are virtual replicas of physical assets, systems, or processes that can be used for simulation, monitoring, and optimization. The combination of AI and IoT with digital twin technology allows industries to create highly accurate models of their operations.
These digital twins can simulate different scenarios, such as equipment failures or production bottlenecks, and provide insights into how to mitigate these issues. By running simulations, industries can test new strategies, optimize processes, and predict outcomes without disrupting actual operations.
For example, in the aerospace industry, digital twins of aircraft engines are used to monitor performance and predict maintenance needs. This technology reduces downtime and ensures that maintenance is carried out only when necessary, rather than on a fixed schedule.
Collaborative AI and Human-Machine Interaction
As AI and IoT become more integrated into industrial production, the nature of human work will evolve. Rather than replacing human workers, AI is expected to collaborate with them, enhancing their capabilities and enabling them to focus on higher-value tasks.
Collaborative robots, or cobots, are designed to work alongside humans, assisting with tasks that require precision, strength, or repetitive actions. These robots can adapt to human workers’ actions and provide support in complex operations.
AI-powered systems can also assist human workers in decision-making by analyzing large datasets and providing actionable insights. For instance, AI can help production managers optimize schedules, allocate resources, and predict demand, enabling more informed and efficient decision-making.
Ethical AI and Responsible Use
As AI becomes more pervasive in industrial production, ethical considerations will play a crucial role in its development and deployment. Industries will need to ensure that AI systems are designed and used responsibly, with a focus on transparency, fairness, and accountability.
For example, AI algorithms used in quality control must be free from biases that could lead to unfair treatment of certain products or suppliers. Industries will also need to consider the ethical implications of AI-driven decision-making, such as the potential impact on employment and the need for human oversight in critical areas.
Regulations and standards for AI and IoT in industrial production are likely to evolve, requiring industries to stay informed and compliant. Responsible AI practices will be essential in building trust and ensuring that these technologies are used for the benefit of all stakeholders.
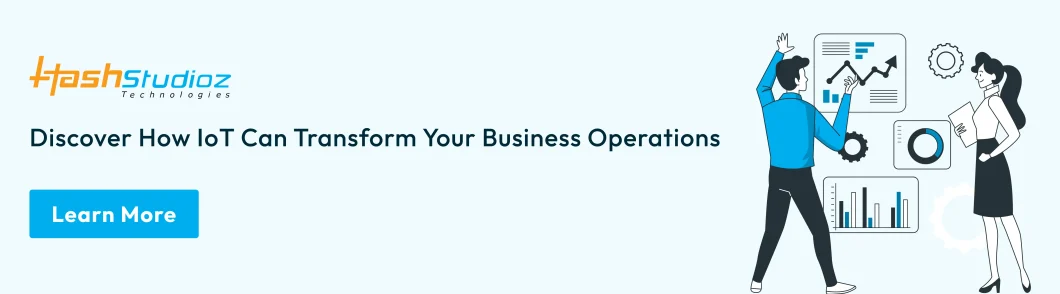
The Role of Human Workers in the AI and IoT-Driven Industry
Upskilling and Reskilling the Workforce
As AI and IoT technologies become more prevalent, the skills required in industrial production will change. Workers will need to be upskilled and reskilled to adapt to new roles that involve interacting with and managing these technologies.
For example, technicians may need training in maintaining and troubleshooting IoT devices, while production managers may need to learn how to interpret data generated by AI systems. Training programs will be essential in ensuring that workers can effectively collaborate with AI and IoT technologies.
Governments, educational institutions, and industries will need to work together to provide accessible training opportunities. Lifelong learning will become increasingly important as technology continues to evolve.
The Human Element in AI-Driven Decision Making
While AI can analyze data and provide recommendations, human judgment will remain crucial in making final decisions. Industries will need to strike a balance between AI-driven automation and human oversight, particularly in areas where ethical considerations or complex trade-offs are involved.
For example, in quality control, AI may identify a batch of products with potential defects. However, the decision to discard, rework, or release the products may require human judgment, considering factors such as customer impact, safety, and cost.
By combining AI’s analytical capabilities with human intuition and experience, industries can make more informed and balanced decisions.
New Roles and Opportunities
The integration of AI and IoT in industrial production will create new roles and opportunities for workers. As routine tasks are automated, workers will be able to focus on more creative, strategic, and value-added activities.
For instance, data analysts will be needed to interpret the vast amounts of data generated by IoT devices, while AI specialists will be required to develop and refine algorithms. Additionally, roles in cybersecurity, ethics, and compliance will become increasingly important as industries navigate the challenges of AI and IoT adoption.
The shift towards AI and IoT-driven production will also open up opportunities for innovation and entrepreneurship. Workers with the right skills will be able to contribute to the development of new products, services, and business models that leverage these technologies.
The Evolution of AI and IoT Technologies
AI Advancements in Machine Learning and Deep Learning
The field of AI is constantly evolving, with advancements in machine learning (ML) and deep learning driving new capabilities. In industrial production, these advancements will enable AI systems to become more accurate, adaptable, and capable of handling complex tasks.
For example, deep learning algorithms can be trained on vast datasets to recognize patterns and make predictions with high precision. In the context of industrial production, this could lead to improvements in predictive maintenance, quality control, and process optimization.
As AI continues to advance, we can expect to see more sophisticated applications in industrial production, such as AI systems that can learn and adapt in real-time, making autonomous decisions with minimal human intervention.
The Proliferation of IoT Devices and 5G Connectivity
The number of IoT devices is expected to grow exponentially, driven by the proliferation of 5G networks. 5G connectivity will enable faster and more reliable communication between IoT devices, supporting the deployment of AI-driven applications in industrial environments.
With 5G, IoT devices can transmit data with low latency, making real-time monitoring and control possible. This will be particularly important in industries that require precise timing, such as manufacturing, logistics, and energy management.
The combination of 5G and IoT will also facilitate the development of new applications, such as remote operation of machinery, augmented reality (AR) for maintenance, and real-time collaboration between geographically dispersed teams.
Integration with Other Emerging Technologies
AI and IoT are likely to be integrated with other emerging technologies, such as blockchain, augmented reality (AR), and quantum computing, to create new possibilities for industrial production.
For example, blockchain technology can be used to secure data generated by IoT devices, ensuring its integrity and authenticity. This is particularly important in supply chain management, where transparency and traceability are critical.
AR can be combined with AI and IoT to provide workers with real-time information and guidance during complex tasks. For instance, AR glasses could display instructions or warnings based on data from IoT sensors, enhancing safety and efficiency.
Quantum computing, while still in its early stages, has the potential to revolutionize AI by solving complex optimization problems that are currently beyond the capabilities of classical computers. In industrial production, quantum computing could be used to optimize supply chains, energy consumption, and production processes.
AI and IoT in Circular Economy and Sustainability
As industries move towards more sustainable practices, AI and IoT will play a key role in enabling circular economy models, where resources are reused, recycled, and regenerated.
AI can optimize resource use by predicting demand, reducing waste, and improving recycling processes. IoT devices can track materials throughout their lifecycle, ensuring that they are reused or recycled efficiently.
For example, in the automotive industry, AI can analyze data from IoT sensors to predict when a vehicle’s components will need to be replaced. This information can be used to schedule maintenance and ensure that parts are recycled or refurbished, rather than discarded.
The combination of AI and IoT will be essential in creating closed-loop systems that minimize waste, reduce environmental impact, and support sustainable industrial practices.
Leading Industrial IoT Platform Providers: Transforming Industry Through Connectivity and Innovation
At HashStudioz Technologies, we provide cutting-edge Industrial IoT (IIoT) platforms designed to transform your industrial operations. Our team excels in developing advanced solutions that enhance operational efficiency, improve system connectivity, and provide actionable insights across your manufacturing processes.
Our comprehensive offerings include scalable IoT platforms, real-time data monitoring tools, and advanced analytics tailored to meet the specific demands of your industry. With our state-of-the-art technologies, you gain enhanced visibility into your operations, predictive maintenance capabilities, and data-driven insights that drive smarter decision-making and operational excellence.
Partner with HashStudioz Technologies to revolutionize your industrial processes and achieve new levels of efficiency and control. Contact us today to discover how our Industrial IoT platforms can optimize your operations and help you achieve your business goals.
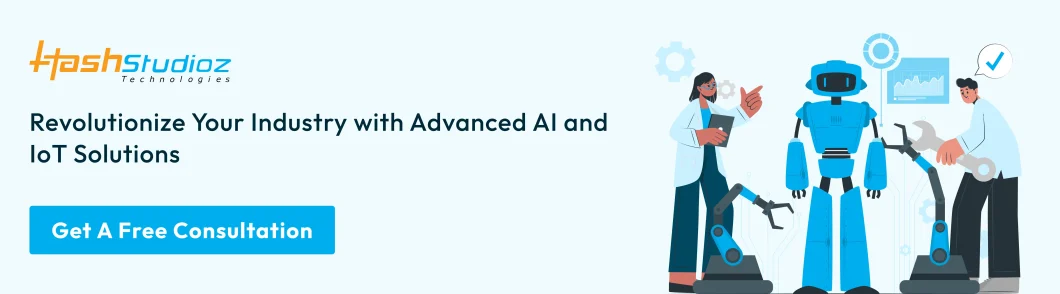
Conclusion:
The integration of AI and IoT is set to reshape industrial production, bringing about a new era of efficiency, innovation, and sustainability. As these technologies continue to evolve, industries will need to adapt, embrace new opportunities, and address the challenges that come with them.
The future of industrial production lies in the seamless collaboration between AI, IoT, and human workers. By leveraging the strengths of each, industries can achieve greater productivity, resilience, and competitiveness in an increasingly complex and dynamic global landscape.
As we move forward, the responsible and ethical use of AI and IoT will be crucial in ensuring that these technologies benefit not only industries but also society as a whole. By focusing on upskilling the workforce, embracing innovation, and prioritizing sustainability, industries can unlock the full potential of AI and IoT, paving the way for a smarter, more connected, and more sustainable future.