Cybersecurity threats are evolving at an alarming rate, with attackers using sophisticated methods to bypass traditional defenses. The growing frequency and complexity of cyberattacks have made conventional methods like rule-based systems and signature-based detection less effective. Cybercrime is projected to cost the world $10.5 trillion annually by 2025, and phishing attacks alone account for 36% of all data breaches globally. Moreover, ransomware attacks increased by 13% in 2023, highlighting the urgent need for advanced defenses. Machine Learning Threat Detection is revolutionizing the cybersecurity landscape. Enter Machine Learning (ML) and AI in Cybersecurity, which provide powerful tools for Machine Learning Threat Detection, allowing organizations to analyze threats, predict malicious behavior, and automate responses. Organizations using these technologies report a 40% faster incident response time and save an average of $3.81 million per breach, making them essential for bolstering cybersecurity measures.
Table of Contents
- The Growing Cybersecurity Landscape
- What Is Machine Learning in Cybersecurity?
- Key Capabilities of Machine Learning in Cybersecurity
- How Machine Learning Works in Threat Detection
- Applications of Machine Learning in Cybersecurity
- Real-World Applications of Machine Learning in Cybersecurity
- Challenges of Using Machine Learning in Cybersecurity
- Future Trends in AI and Machine Learning in Cybersecurity
- Case Study: Using Machine Learning for Threat Detection
- The Economic Impact of AI in Cybersecurity
- Take Your Cybersecurity to the Next Level with HashStudioz Technology
- Why Choose HashStudioz Technology for Cybersecurity?
- Why Hiring Expert Developers at HashStudioz Maximizes ROI
- Conclusion
- FAQ: Machine Learning in Cybersecurity
The Growing Cybersecurity Landscape
Cyber Threat Statistics
- Increased Attacks: Cybersecurity Ventures predicts that cybercrime will cost the world $10.5 trillion annually by 2025.
- Complex Threats: Zero-day vulnerabilities and polymorphic malware are becoming more prevalent, making detection harder.
- Data Breaches: The average cost of a data breach in 2023 was $4.45 million, as reported by IBM.
This landscape underscores the need for advanced, adaptive technologies like machine learning to stay ahead of attackers.
What Is Machine Learning in Cybersecurity?
Machine learning is a branch of artificial intelligence that enables systems to learn from data and improve over time without explicit programming. In cybersecurity, ML algorithms process vast datasets to detect patterns, anomalies, and irregularities that indicate potential threats.
Unlike static security tools, ML systems adapt and evolve as they learn from new data, making them particularly effective against dynamic and unknown attack vectors.
Key Capabilities of Machine Learning in Cybersecurity
1. Behavioral Analysis
Machine learning models analyze user, network, and application behavior to identify deviations from the norm.
- Example: Identifying a user account suddenly attempting to access sensitive files outside of usual work hours.
2. Automated Threat Detection
ML systems can autonomously detect phishing emails, malware, or intrusion attempts, reducing reliance on manual interventions.
3. Predictive Threat Intelligence
By analyzing historical attack data, ML can predict potential attack vectors, enabling proactive defenses.
4. Incident Response Automation
Machine learning models can automate incident responses, such as isolating compromised devices or flagging suspicious IP addresses.
How Machine Learning Works in Threat Detection
1. Data Collection and Integration
Machine learning systems gather data from multiple sources, including:
- Network traffic logs
- Firewall alerts
- Endpoint security tools
- Cloud-based systems
This diverse data provides the foundation for accurate threat analysis.
2. Feature Extraction
The raw data is processed to extract meaningful features, such as:
- Unusual login patterns
- Data transfer anomalies
- Sudden spikes in network traffic
These features help the ML model focus on critical aspects of cybersecurity threats.
3. Training the Model
ML models are trained using supervised, unsupervised, or reinforcement learning techniques.
- Supervised Learning: Trains the model using labeled datasets of known threats and safe activities.
- Unsupervised Learning: Identifies anomalies in unlabeled data to detect unknown threats.
- Reinforcement Learning: Improves the model’s decision-making process over time through feedback loops.
4. Threat Detection and Classification
The trained model classifies threats based on severity and type, such as phishing, malware, or denial-of-service (DoS) attacks.
Zero Trust Security in IoT: A Comprehensive Guide to Implementing Principles in IoT Solutions
Applications of Machine Learning in Cybersecurity
1. Phishing Detection
Phishing emails remain a leading cause of cyber breaches. ML models analyze:
- Email headers
- Links and attachments
- Writing patterns and language tone
This approach enables the detection of phishing attempts, even those using novel techniques.
- Stat: Verizon reports that phishing was responsible for 36% of breaches in 2023.
2. Malware Analysis
Machine learning identifies malware by analyzing behavioral patterns instead of relying solely on signatures.
- Example: ML detects ransomware by monitoring encryption activities and unusual file access patterns.
- Stat: ML-based tools can identify up to 99% of malware, including previously unseen variants.
3. Network Intrusion Detection
ML-powered intrusion detection systems (IDS) monitor network traffic for anomalies.
- Example: Spotting an attacker attempting to exfiltrate sensitive data.
- Benefit: Real-time analysis minimizes damage from breaches.
4. Fraud Prevention
Financial institutions use ML to detect fraudulent transactions. Algorithms analyze transaction patterns, user locations, and device data to flag suspicious activity.
- Stat: The global fraud detection market is expected to reach $40.8 billion by 2027, according to MarketsandMarkets.
5. User Behavior Analytics (UBA)
ML examines user behavior to detect insider threats.
- Example: An employee downloading large volumes of sensitive data without authorization triggers an alert.
Real-World Applications of Machine Learning in Cybersecurity
1. Microsoft Defender
Microsoft uses ML to detect ransomware by analyzing encryption and file modification patterns. Its systems block millions of threats daily.
2. Darktrace
Darktrace, an AI-driven cybersecurity platform, employs ML to detect and respond to cyber threats autonomously.
- Example: It uses unsupervised learning to identify novel attacks, even those bypassing traditional defenses.
Challenges of Using Machine Learning in Cybersecurity
1. Adversarial Attacks
Attackers may manipulate ML models by feeding them false data, leading to incorrect predictions.
- Example: An attacker tricks a model into classifying malware as legitimate software.
2. High Computational Costs
Training and deploying ML models require substantial computational resources, which may not be feasible for small businesses.
3. Bias in Data
If the training data is biased or incomplete, the ML model may produce inaccurate results, leaving systems vulnerable.
4. Complexity
Implementing machine learning in cybersecurity requires skilled professionals who can build, train, and maintain these systems.
Future Trends in AI and Machine Learning in Cybersecurity
1. AI-Powered Security Operations Centers (SOCs)
AI and ML will play a central role in SOCs by automating routine tasks, analyzing large datasets, and prioritizing critical incidents.
2. Zero Trust Architecture
ML will support zero trust models by continuously verifying user identities and monitoring access patterns.
3. IoT Security Enhancements
As IoT devices grow, ML will help detect vulnerabilities and prevent attacks on connected systems.
- Stat: By 2030, over 29 billion IoT devices are expected to be operational, according to Ericsson.
4. Predictive Cybersecurity
ML models will evolve to predict attacks before they occur, enabling more proactive defense strategies.
Case Study: Using Machine Learning for Threat Detection
Problem
A global financial institution faced frequent phishing and malware attacks, compromising sensitive customer data.
Solution
The institution deployed a machine learning-based cybersecurity solution.
- An ML algorithm analyzed over one million emails daily.
- It flagged phishing attempts based on behavioral patterns and language analysis.
Outcome
- Phishing attacks were reduced by 80%.
- False positives decreased by 50%, saving time and resources.
IoT in Industrial Automation: Enhancing Operational Efficiency Across Industries
The Economic Impact of AI in Cybersecurity
Investing in machine learning-based cybersecurity solutions yields significant economic benefits.
- Stat: Organizations using AI and ML reduced their average breach costs by 20%, as per IBM.
- Automated responses save thousands of work hours annually.
Take Your Cybersecurity to the Next Level with HashStudioz Technology
In an era where cyber threats evolve daily, safeguarding your business is no longer optional it’s essential. The rising sophistication of attacks, such as phishing, ransomware, and advanced persistent threats, demands proactive and intelligent defenses. At HashStudioz Technology, we leverage the power of AI in Cybersecurity and Machine Learning solutions to deliver state-of-the-art protection tailored to your unique needs.
Our solutions go beyond traditional methods, offering real-time threat detection, anomaly analysis, and automated responses to mitigate risks before they impact your business. With Machine Learning algorithms, our systems continuously learn from data, enabling us to stay ahead of attackers by identifying and neutralizing emerging threats.
Why Choose HashStudioz Technology for Cybersecurity?
When it comes to protecting your business from the increasing complexity and frequency of cyberattacks, choosing the right partner is crucial. HashStudioz Technology stands out as a leading provider of cybersecurity solutions, offering cutting-edge tools and tailored strategies to ensure your organization remains resilient against all forms of cyber threats. Here’s a detailed explanation of why we are the ideal choice:
Our Services
1. Advanced AI & ML Integration
Cyber threats are becoming more sophisticated, requiring intelligent systems to stay ahead. At HashStudioz Technology, we leverage Artificial Intelligence (AI) and Machine Learning (ML) to create advanced security frameworks that:
- Detect complex threats: Our ML algorithms analyze large datasets in real-time to identify anomalies and detect even the most intricate attack patterns, such as zero-day exploits and advanced persistent threats (APTs).
- Minimize false positives: Traditional security systems often flag non-threatening activities as potential risks, overwhelming teams with alerts. Our AI-driven tools reduce these false positives, allowing your security team to focus on genuine threats.
Example: One of our solutions enabled a financial institution to reduce false alerts by 50% while accurately identifying fraudulent transactions.
2. Automated Incident Response
Manual threat response is often time-consuming and prone to delays, which can amplify the damage caused by cyberattacks. Our ML-powered systems automate the incident response process by:
- Analyzing threats in real time: When a potential attack is detected, our tools immediately assess its severity and scope.
- Triggering automated actions: From isolating infected systems to blocking malicious IPs, our solutions take swift action to neutralize threats.
- Improving response times: Organizations using our automated response solutions report a 40% reduction in incident resolution times compared to manual methods.
For example, a retail client experienced a ransomware attack, but our automated response system contained the threat within minutes, saving critical data and preventing downtime.
3. Custom Security Solutions
Every business faces unique challenges based on its industry, size, and operations. At HashStudioz Technology, we don’t offer one-size-fits-all solutions. Instead, we:
- Assess your needs: Conduct a thorough audit of your current systems, vulnerabilities, and potential risks.
- Develop tailored strategies: Create customized solutions aligned with your industry’s specific requirements, whether it’s regulatory compliance in healthcare or fraud prevention in e-commerce.
Example: For an IoT-focused client, we developed a bespoke solution to secure device communications and prevent unauthorized access, ensuring seamless operations in a highly connected environment.
4. Cost Savings
Data breaches can have devastating financial implications, including legal fees, reputational damage, and lost business. Organizations leveraging HashStudioz Technology’s AI-driven cybersecurity solutions benefit from:
- Reduced breach costs: According to IBM, companies using AI in cybersecurity save an average of $3.81 million per breach compared to those without.
- Efficient resource allocation: By automating threat detection and response, you reduce dependency on manual labor, cutting operational costs.
For instance, a global logistics company saved millions by deploying our proactive threat detection systems, which prevented several attempted breaches in a year.
Why Hiring Expert Developers at HashStudioz Maximizes ROI
- Tailored Solutions: We don’t rely on generic themes. Every website we build is uniquely designed for your brand.
- Enhanced Performance: Our developers implement speed optimization techniques to improve user experience and conversion rates.
- Scalability: We design websites that grow with your business, accommodating future expansions.
- Robust Security: With advanced measures, we protect your website against cyber threats, ensuring peace of mind.
- Cost Efficiency: By getting it right the first time, we reduce long-term maintenance costs, saving you money.
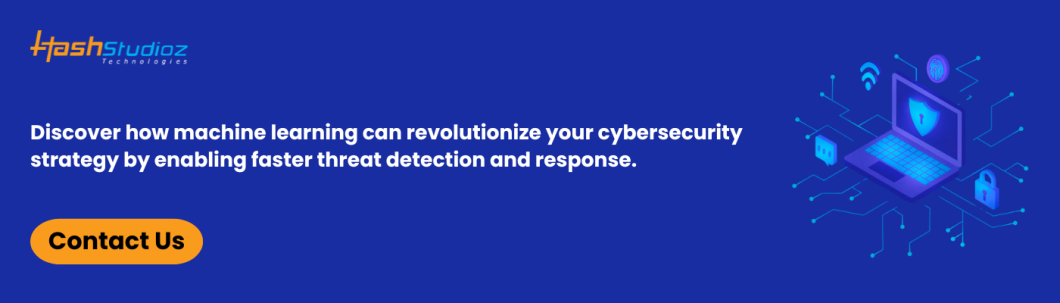
Conclusion
Machine learning is reshaping cybersecurity by enabling advanced threat detection, rapid responses, and predictive intelligence. Its ability to analyze vast datasets, identify anomalies, and automate responses makes it indispensable in combating modern cyber threats. While challenges like adversarial attacks and high resource requirements exist, the benefits far outweigh the limitations. Businesses must invest in skilled professionals, robust ML models, and high-quality data to harness its full potential.
By adopting AI in Cybersecurity, organizations can secure their systems, protect sensitive data, and maintain trust in an increasingly digital world. With its rapid evolution, machine learning will remain a cornerstone of cybersecurity strategies for years to come.
FAQ: Machine Learning in Cybersecurity
Q1: What is the role of machine learning in cybersecurity?
A: Machine learning enhances cybersecurity by analyzing large datasets to detect patterns, anomalies, and potential threats. It helps in identifying unknown attack vectors, automating threat responses, and improving overall security measures.
Q2: How does machine learning detect threats?
A: Machine learning models use algorithms to analyze behavioral patterns, network traffic, and system logs. By identifying deviations from normal behavior, it can flag potential threats like phishing, malware, or insider attacks.
Q3: Can machine learning prevent all cyberattacks?
A: No, while machine learning significantly improves threat detection and response, it cannot prevent all attacks. Sophisticated threats like adversarial attacks may still bypass defenses. ML works best when integrated with other cybersecurity measures.
Q4: How is machine learning different from traditional cybersecurity methods?
A: Traditional methods rely on predefined rules and signatures, which may fail against novel threats. Machine learning adapts and learns from new data, enabling it to detect previously unseen attack vectors.
Q5: How does machine learning improve response time in cybersecurity?
A: ML automates threat identification and response processes, reducing the need for manual intervention. This leads to faster mitigation of threats, minimizing potential damage.